What is a Linear Model?
Elevate your expertise in linear models and learn more hierarchical linear modeling (HLM) and structural equation modeling (SEM).
What is a Linear Model? Heading link
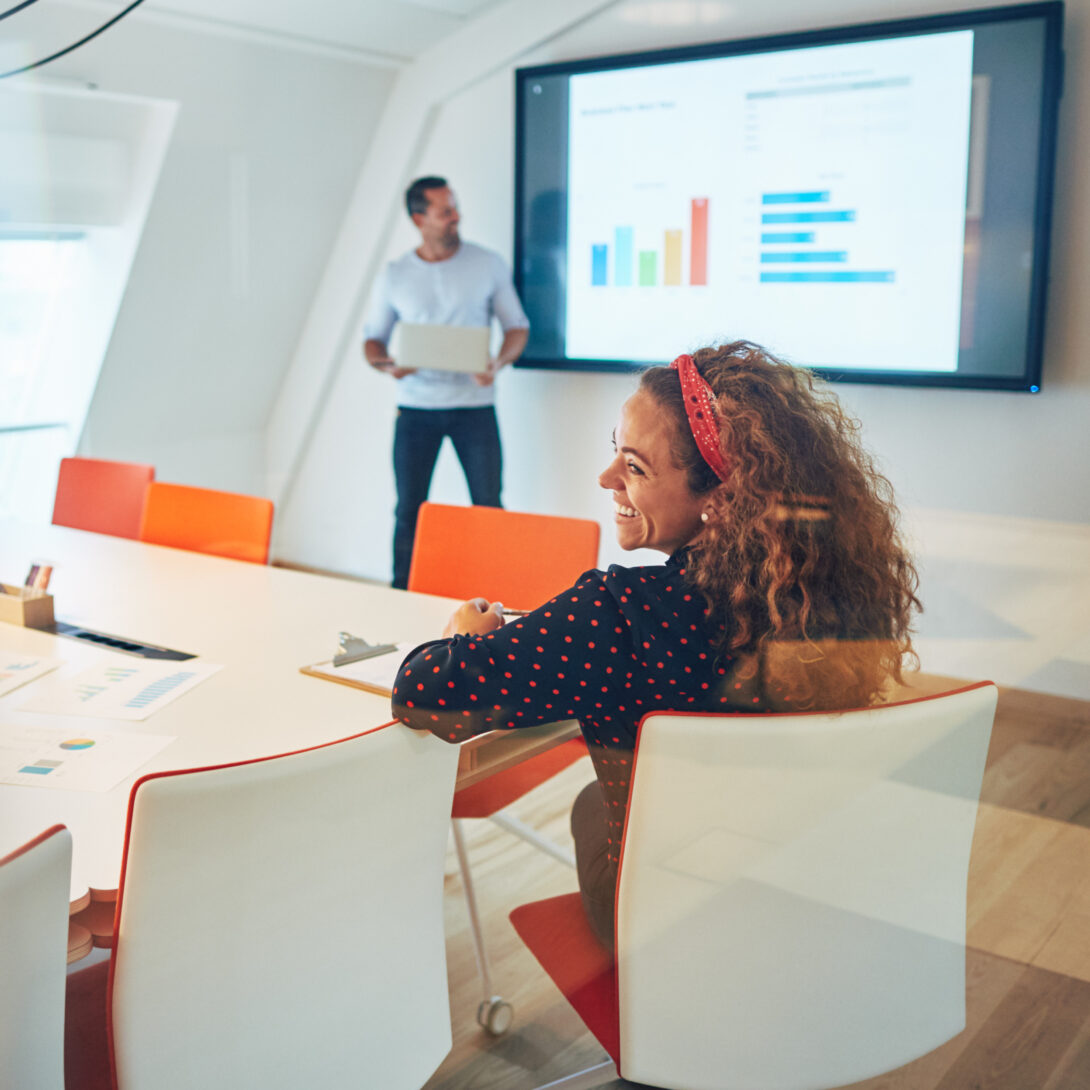
Linear models are a class of equations that describe relationships between two quantities exhibiting a constant rate of change. They serve as analytic tools aiding researchers in understanding relationships within data and are widely employed in various fields such as economics, psychology, biology, and more.
In this article, we will explore the fundamentals of linear models and the distinction between two advanced techniques, hierarchical linear modeling (HLM) and structural equation modeling (SEM).
Understanding Linear Models
Linear models assume a linear relationship between independent and dependent variables. The term “linear” means the connection between input and output forms a straight line on a graph. For example, when estimating a house’s price based on its size, a simple linear model would look like this:
Price = Weight × Size + Intercept
“Weight” shows how much size affects the price, and “intercept” is a constant. The model figures out the best values for the “weight” and “intercept” from data points to make accurate predictions for new data.
There are various types of linear models, each suited for different purposes in statistical modeling. Some common types include simple linear regression, multiple linear regression, hierarchical linear models (HLM), and structural equation models (SEM), etc. While SEM focuses on relationships among variables, HLM focuses on the effects of different levels of analysis on an outcome. Both methods are powerful tools for analyzing multivariate data and can provide valuable insights in their respective domains.
Hierarchical Linear Modeling (HLM):
Hierarchical linear modeling (HLM), also known as multilevel modeling, is an extension of linear models designed to handle nested or hierarchical data structures. This approach is particularly useful when data is organized hierarchically, like students within schools or employees within companies.
HLM allows for the modeling of both within-group (individual-level) and between-group (group-level) variations. It recognizes that observations within the same group may be correlated, and it accommodates this correlation in the analysis. HLM is beneficial in capturing the complexity of real-world data where observations are not independent.
Structural Equation Modeling (SEM):
Structural equation modeling (SEM) is a statistical technique that extends beyond linear models. SEM enables researchers to examine complex relationships among continuous and categorical variables, incorporating both observed and latent variables. SEM represents the relationships between variables through a system of structural equations.
Dr. Ting Dai, UIC Assistant Professor for the online Measurement, Evaluation, Statistics, and Assessment (MESA) programs at the University of Illinois Chicago (UIC), emphasizes the importance of learning structural equation modeling.
“To me, SEM is not just a statistical method, but it is a way of thinking that opens up your mind in terms of how to approach educational research and psychological research,” said Dr. Dai. “Problems that we are faced with in the real-world are not in the vacuum of a lab. We need to look at a lot of variables that are intricate and are interconnected and SEMs help us to do that.”
SEM is widely used in various disciplines, including psychology and social sciences, to test and refine theoretical models.
An Education in Linear Models
Elevate your expertise in linear models through advanced courses in HLM and SEM offered by UIC’s online Master of Education and Certificate in MESA programs. The program’s advanced courses in HLM and SEM ensure that students not only gain a comprehensive understanding of these sophisticated statistical methodologies but also acquire practical skills to apply them in real world scenarios.
The EPSY 584: Hierarchical Linear Models course covers regression, assumptions, parameter estimation, and hypothesis testing, including practical examples and lab sessions. The EPSY 587: Structural Equation Modeling course delves into SEM for cross-sectional, longitudinal, and experimental data analysis, emphasizing model specification, model evaluation and selection, data setup, variable coding, and the use of Mplus for latent variable modeling.
“Since we launched the online MESA program in 2009, there’s been maybe 5-6 similar programs that have launched,” said MESA Professor and Program Director Dr. Everett Smith. “However, when we review these programs’ curriculums, we are still the only programs offering these advanced courses.”
MESA graduate Jean-Paul Cruz-Walker highlights the real-world benefits of these courses in his career as an Institutional Research and Educational Assessment Analyst.
“The exposure to the higher-up classes, like HLM, was great,” said Jean-Paul. “I really appreciated getting more exposure to the advanced statistical techniques and my boss, who graduated from the UIC MESA certificate program, was confident in my skills from her experience with the quality of the MESA professors.”
Whether unraveling the threads of hierarchical structures or exploring intricate relationships, these advanced statistical tools empower researchers to extract meaningful insights from their data. Talk to an enrollment specialist to learn more about UIC’s online MESA programs. This 100% online program allows flexibility, convenience, and autonomy for both students and instructors.